Data has transformed the world of computing, evolving from simple data processing to complex artificial intelligence (AI) and machine learning systems. This journey has been marked by significant milestones, each bringing new capabilities, challenges, and opportunities. Let’s explore how data and its processing evolved from the 1950s to the present, shaping the landscape we see today in AI, cloud computing, and big data analytics.
1950s: Early Data Processing
The 1950s marked the beginning of electronic data processing, a foundational period for the entire data industry. Back then, computers were massive machines, operated primarily by government institutions and large corporations. Data processing was limited and focused on handling basic calculations and storage. The emphasis was on using data to improve business efficiency and streamline administrative tasks, laying the groundwork for future data applications.
Key Takeaway: The 1950s introduced the world to the concept of electronic data processing, setting the stage for data-driven operations in various sectors.
1960s: Emergence of Computers
The 1960s witnessed the proliferation of computers, with an increasing number of businesses adopting this revolutionary technology. Mainframe computers became more accessible, though still primarily for large enterprises due to cost and complexity. With the rise of computing power, data processing capabilities improved, and databases began to take shape. This era focused on data storage and retrieval, enabling companies to organize and manage large amounts of information more effectively.
Key Takeaway: The 1960s saw data processing expand beyond government use, with more businesses adopting computer technology for data management.
1970s: Rise of Relational Databases
In the 1970s, data management saw a breakthrough with the invention of relational databases. IBM’s Structured Query Language (SQL) and the development of the relational database management system (RDBMS) allowed for structured data storage and retrieval. This approach simplified data access, enabling businesses to efficiently manage and query data. As relational databases gained popularity, they became the standard for data storage, shaping the way data would be managed in the decades to come.
Key Takeaway: The 1970s established relational databases, revolutionizing data management and making data more accessible for business applications.
1980s: Personal Computers and Client-Server Computing
The 1980s brought about personal computers (PCs) and the client-server computing model, marking a shift toward decentralization. With PCs in workplaces and homes, more people began working with data directly. Client-server computing allowed data to be processed on local machines while leveraging centralized databases, enhancing collaboration and accessibility. This era paved the way for more user-friendly data applications and expanded data processing to a broader audience.
Key Takeaway: The 1980s democratized data access through personal computers and client-server architectures, bringing data processing capabilities closer to individual users.
1990s: Internet Revolution
The 1990s introduced the internet, a transformative technology that reshaped data handling forever. Data could now be transmitted globally, allowing for real-time access and sharing of information. This era also saw the growth of web-based applications, e-commerce, and online data-driven services. With the rise of the internet, data became more valuable, leading to the development of data-centric applications that could scale to serve millions of users.
Key Takeaway: The internet revolution of the 1990s expanded data access globally, ushering in an era of connectivity and real-time data interaction.
2000s: Big Data Emergence
With the explosion of internet usage, social media, and mobile devices, the 2000s introduced the concept of big data. Massive amounts of unstructured data were generated, far exceeding traditional data processing capabilities. Technologies like Hadoop and NoSQL databases emerged to handle the vast volumes, velocity, and variety of data. This shift redefined data analytics, opening doors for advanced data processing techniques that could uncover insights from previously unusable data.
Key Takeaway: The 2000s saw the rise of big data, necessitating new technologies to process, analyze, and extract insights from unprecedented data volumes.
2010s: Big Data Analytics and Cloud Computing
The 2010s built on big data concepts by integrating big data analytics and cloud computing. Cloud platforms allowed companies to store and process vast amounts of data without needing on-premises infrastructure. Simultaneously, advancements in big data analytics enabled organizations to derive actionable insights from complex datasets, transforming industries from healthcare to finance. This decade marked a shift toward data-driven decision-making at scale.
Key Takeaway: The 2010s introduced scalable data storage and processing through cloud computing, enabling large-scale data analytics and democratizing access to data insights.
2020s: AI and Machine Learning
Today, in the 2020s, we are witnessing the convergence of big data, AI, and machine learning. AI technologies leverage big data to train models, enabling machines to identify patterns, predict outcomes, and make decisions autonomously. Machine learning algorithms, powered by vast datasets, have given rise to applications like natural language processing, image recognition, and predictive analytics. The integration of AI into data processing marks a new era of innovation, with applications impacting every industry.
Key Takeaway: The 2020s represent a new frontier in data, where AI and machine learning leverage big data for intelligent, autonomous decision-making.
Concluding Thoughts: From Data Processing to Intelligent Systems
The journey from simple data processing in the 1950s to advanced AI systems in the 2020s highlights the incredible evolution of data technologies. Each decade brought new advancements, making data more accessible, scalable, and intelligent. Today, data is not just an operational asset; it’s a strategic resource driving innovation and shaping the future of industries worldwide. As we look forward, the integration of data, AI, and emerging technologies promises even more groundbreaking transformations in how we understand and leverage information.
Read Also:
- Human Brain vs. Neural Networks: A Technological Perspective
- Understanding Artificial Intelligence: A Human-Centric Overview
- Addressing AI Risks: Achieving the AI Risk Management Professional Certification
- Mastering Scaled Scrum: Earning the Scaled Scrum Professional Certification
- Strengthening Agile Leadership: Achieving the Scrum Master Professional Certificate
- Advancing My Expertise in AI: Earning the CAIEC Certification
- Achieving the CAIPC Certification: Advancing My AI Expertise
Subscribe to the GnoelixiAI Hub newsletter on LinkedIn and stay up to date with the latest AI news and trends.
Subscribe to my YouTube channel.
Reference: aartemiou.com (https://www.aartemiou.com)
© Artemakis Artemiou
Rate this article:
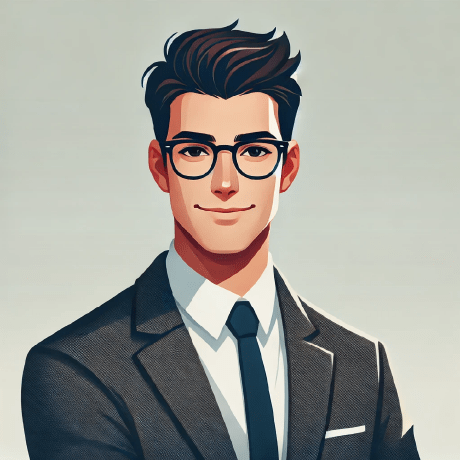
Artemakis Artemiou is a seasoned Senior Database and AI/Automation Architect with over 20 years of expertise in the IT industry. As a Certified Database, Cloud, and AI professional, he has been recognized as a thought leader, earning the prestigious Microsoft Data Platform MVP title for nine consecutive years (2009-2018). Driven by a passion for simplifying complex topics, Artemakis shares his expertise through articles, online courses, and speaking engagements. He empowers professionals around the globe to excel in Databases, Cloud, AI, Automation, and Software Development. Committed to innovation and education, Artemakis strives to make technology accessible and impactful for everyone.